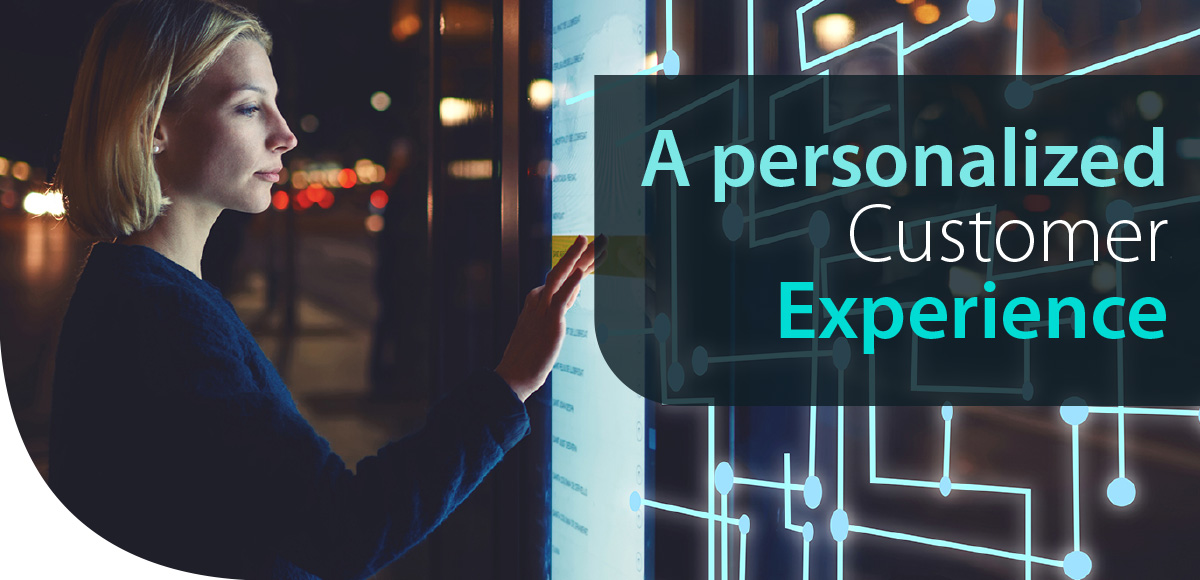
What is an AI Recommendation Engine?
An AI Recommender System suggests products or services based on user data, which includes their preferences, past purchases, demographic information, and the behavior of similar users. It brings a convenient way for e-commerce companies, retail stores and even banks to offer personalized solutions to their customers. The type of AI recommendations offered depends on the data available, the ability to scale, and the transparency of the recommendations.
Recommendation systems are becoming increasingly popular as they help users navigate the overwhelming amount of digital information and products.
A recommendation engine uses Machine Learning and Big Data to suggest additional products or services to consumers. These systems are trained to understand user preferences and characteristics, including impressions, clicks, likes, and purchases. Content and product providers favor these systems as they can drive consumers to a variety of products or services that interest them, from electronics to movies to yoga classes to catering services, etc...
In a world where there is a lot of information and product overload, recommendation engines provide an efficient way for companies to provide consumers with personalized information and solutions. As users become more comfortable with digital recommendations, recommender systems are becoming the primary way for them to discover products and services that they might otherwise have not found on their own.
80% of customers are more likely to purchase a product or service from a brand that provides personalized experiences *
*Epsilon
Types of Recommendation Systems
There are three main types of recommendation systems:
- Content-based filtering uses a user's history and interactions to make recommendations based on established patterns in their choices or behaviors. Higher accuracy depends on the quantity of information that the user provides.
A type of recommendation system that falls under content-based filtering is known as a case-based recommender. This system recommends items based on their similarities and are commonly used in e-commerce to improve the quality of their recommendations. An example of this approach is suggesting “products similar to this” or “products you might also like”.
- Collaborative filtering collects information from many other users to derive suggestions for you based on other users with similar tastes or situations.
‘Next buy’ recommendations are an example of collaborative filtering. This method utilizes the resemblance of user preference patterns, based on past interactions between users and items. By analyzing these interactions, recommender algorithms can learn to make predictions about future interactions. The concept is that if certain individuals have made comparable choices and transactions before, such as selecting a clothing item, then there is a strong chance that they will have similar preferences for future options.
Collaborative filtering typically provides more accurate recommendations compared to content-based filtering, however, it is particularly weak when the available data is not sufficient.
- Knowledge-based systems make recommendations based on an influence about a user's needs and based on a degree of domain expertise and knowledge and not necessarily on the interaction history of a user. Where the purchase history of the users is smaller, these systems aim to capture the knowledge of human experts to support decision-making.
Knowledge-based recommender systems are particularly appropriate for intricate fields in which items are not frequently bought, such as real estate and automobiles. These systems evaluate item knowledge, including characteristics, explicitly requested user preferences, and recommendation criteria, to generate suggestions. The effectiveness of the model is evaluated by the usefulness of the recommended item to the user.
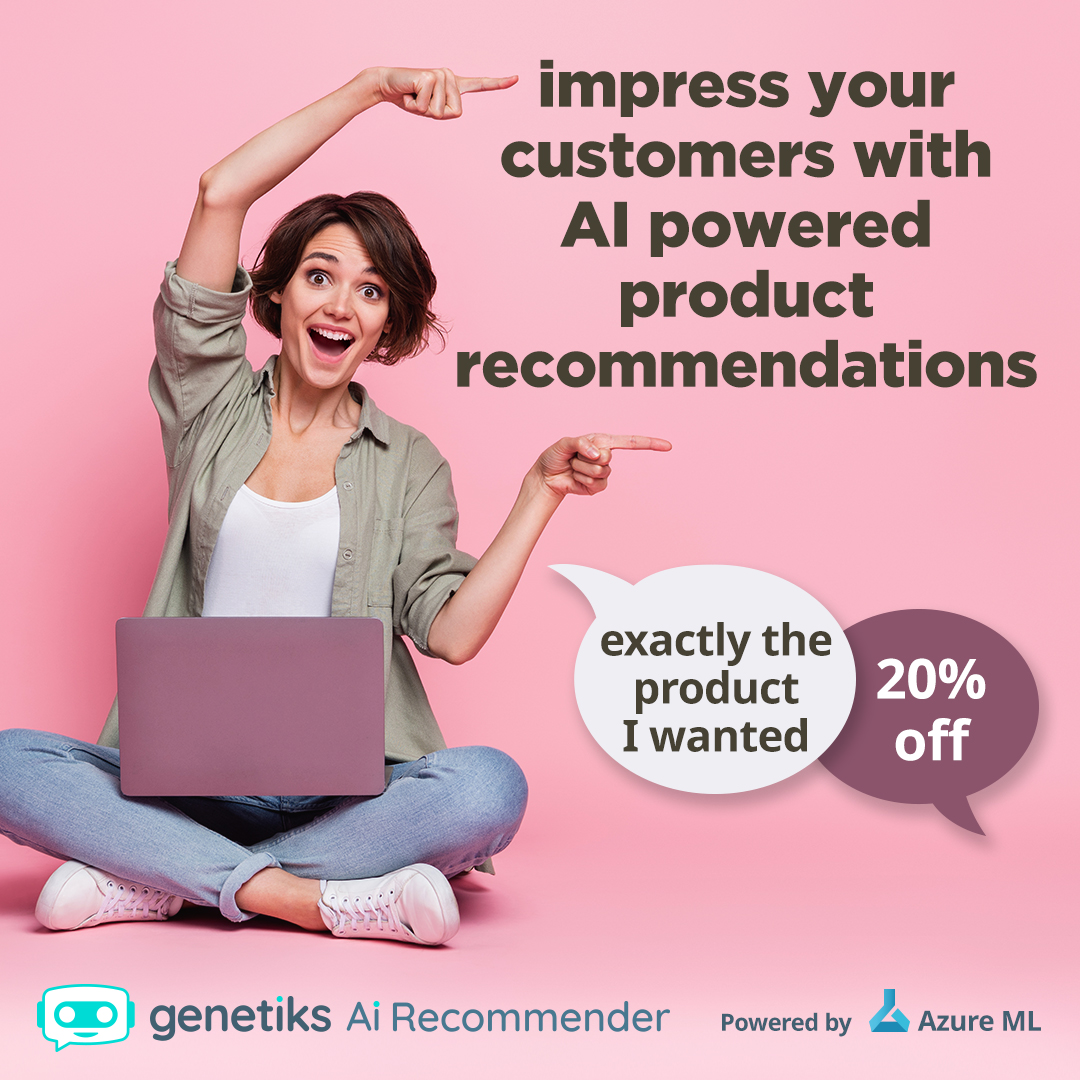
Hybrid recommender systems combine the advantages of these types to create a more comprehensive recommending system.
The Wide and Deep recommender is a typical hybrid recommender system, as it uses both the content-based and collaborative filtering approaches described above.
As a result, Wide and Deep recommendations use the historical behavior of the user and generalize them through “inspiration” from similar users, so they also recommend relevant products never bought by the user before.
How does a Recommendation Engine Work?
1- Data Collection:
Typically, recommendation systems collect two types of information: implicit and explicit.
Implicit data includes data gathered from user actions such as search history, clicks, items added to carts, and order history.
Explicit data is collected from customer conduct, such as reviews and ratings, likes and dislikes, and comments.
Recommendation engines also utilize customer data such as demographics (age, gender) and psychographics (interests, values) to identify similar patterns between various customers, as well as feature data (genre, item type) to identify similarities between products.
2- Data Storage:
Scalable storage that can keep up with your data growth is necessary. As time passes, the volume of data will only increase, therefore having various kinds of storage for each data set that are scalable and dependable is important.
3- Data Analysis:
Following that, the data is processed and examined in various ways: real-time analysis (data is processed as it is created), batch analysis (data is processed periodically), and near-real-time analysis: Data is processed in minutes after it’s gathered.
4- Recommendation Generation:
In the final step, the data is refined utilizing various matrices or mathematical rules and formulas based on the recommendation filtering model. The recommendations are the outcome of this filtering.
71% of users uninstall a mobile app due to irrelevant notifications *
*Dot Com Infoway (DCI)
Recommendation engines offer a significant marketing advantage, as they can help increase revenue, conversions, click-through rates, and even customer satisfaction. Essentially, these engines use machine learning to suggest relevant products or services to users, and you likely interact with them every day through various applications, such as dating apps, movie suggestions, and product recommendations.
As brands gather more data on user behavior, recommendation engines become more valuable in providing effective product suggestions. By driving user engagement and encouraging users to return to a particular store or service, these recommendations play a crucial role in maintaining customer loyalty.
While the preceding discussion has only covered the fundamentals of recommendation engines, this subject is much broader and multifaceted. Nevertheless, we hope that this introduction was helpful.
Now it's time to explore how an AI Recommender Engine can help optimize your company’s conversion rates and retention rates.