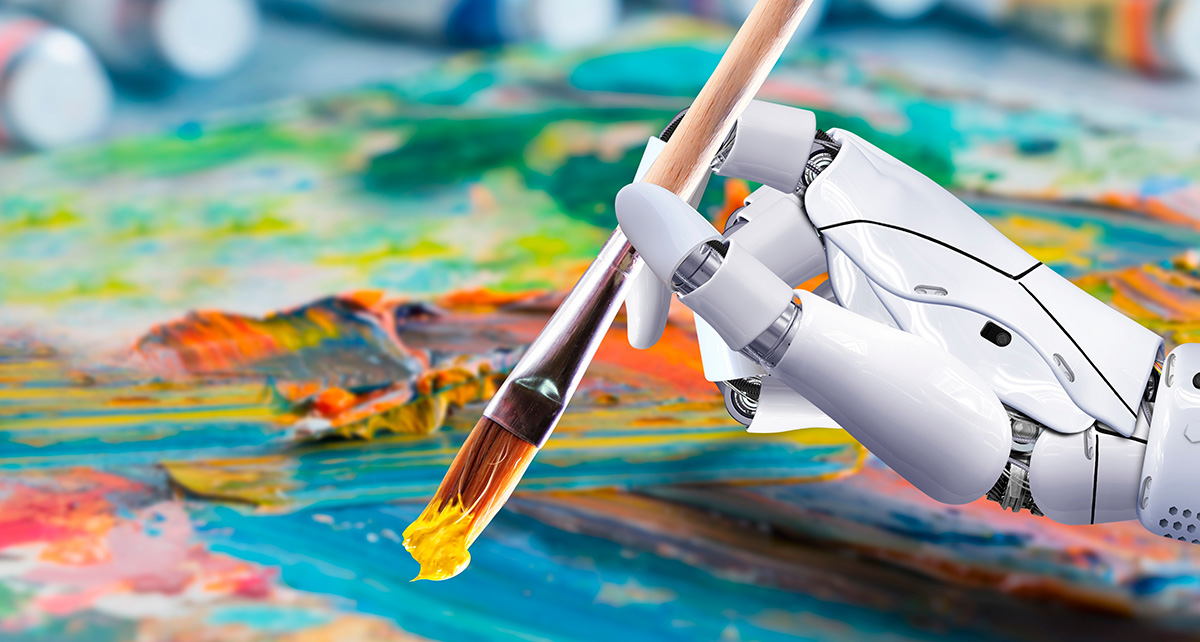
What is Generative AI?
Generative AI, also known as genAI, refers to a type of Artificial Intelligence capable of crafting diverse content like text, images, videos, and audio, showcasing human-like creativity. Recent strides in this field, marked by breakthroughs like ChatGPT (an AI-powered chatbot developed by OpenAI) and Midjourney, have elevated generative AI's capabilities, making it invaluable in sectors such as gaming, entertainment, and product design.
This technology swiftly generates content based on various inputs, like text, images, sounds, animations, or 3D models, using neural networks. Notable examples include GPT-3.5 and Stable Diffusion, catering to language-based tasks and generating photorealistic images, respectively.
Generative AI distinguishes itself by focusing on content creation, setting it apart from other AI forms dedicated to tasks like data analysis or autonomous vehicle control.
63 Generative AI use cases, spanning 16 business functions, could deliver a total value of $2.6 trillion to $4.4 trillion in economic benefits annually. *
* McKinsey Digital
How did Generative AI Technology Evolve?
Initially, simple models like Markov chains were used for tasks such as predicting the next word in a sentence. However, these models had limitations due to their simplicity and inability to generate realistic text.
In 2013, Variational Autoencoders (VAEs) were the first deep generative models capable of producing realistic images and speech by creating new variations of different data types. Following VAEs, generative adversarial networks and diffusion models emerged, focused on generating data that closely resembled real-world information.
In 2017, transformers entered the scene, revolutionizing AI research. They integrated an encoder-decoder architecture with an attention mechanism, enhancing the training process for language models.
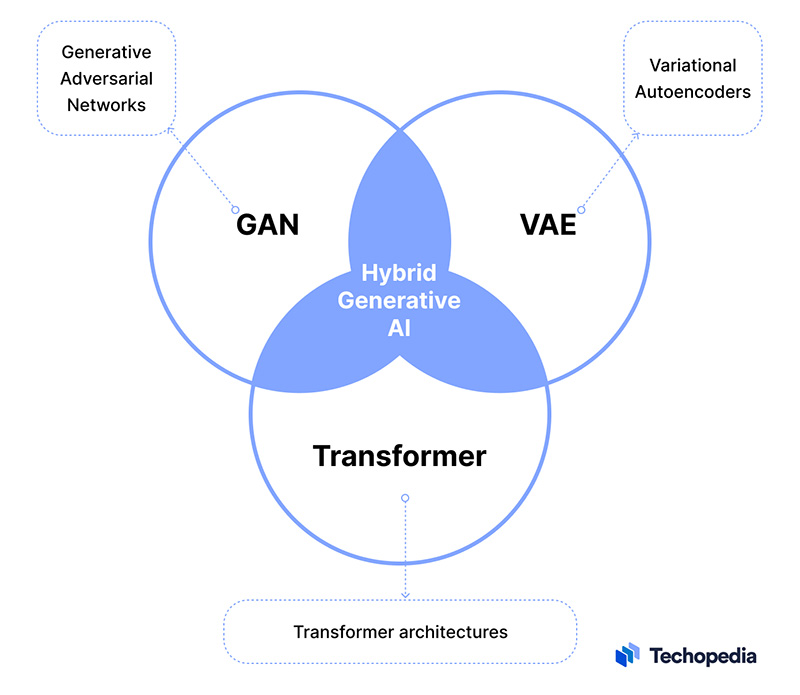
Source: Techopedia
The evolution continued with the emergence of large and complex models like ChatGPT. Trained on extensive internet text, ChatGPT understands text patterns, proposing what might come next based on learned statistical chunks. These advancements hold the potential to transform various industries, from sales and marketing to software development.
Applications in Image Generation
Generative AI, including models like VAEs, GANs, and Stable Diffusion, produce images with real-world visuals. Image Generation finds applications in various fields, such as data augmentation for improving machine learning models, creating art, generating product images, and more.
Platforms like MidJourney and DALL-E 2, trained on billions of internet images, have gained popularity for their success in generating realistic images, connecting words to visual elements and allowing users to generate imagery in various styles. Generative AI's image-related applications extend to creating 3D images, avatars, videos, graphs, and other illustrations.
Various alternatives, such as Bing's Image Generator, offer advanced capabilities. These models mark a shift from traditional discriminative machine learning to generative models that learn features and relationships, enabling the recreation of new images not in the training set.
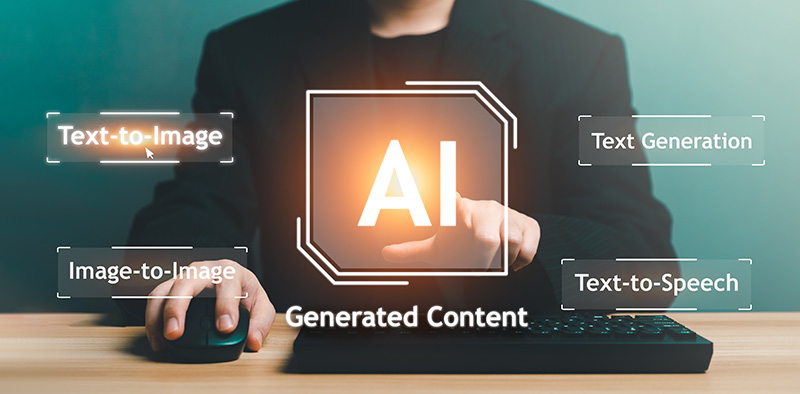
Applications in Text Generation
Text Generation, that involves using machine learning models such as Markov Chains, Recurrent Neural Networks (RNNs) and Transformers, has witnessed remarkable advancements in recent years. Notably, ChatGPT gained widespread popularity in November 2022, using OpenAI's GPT-3.5 implementation. This chatbot stands out by incorporating the history of its conversation with users, generating human-like responses and creating a more natural and interactive experience.
Large Language Models (LLMs), focus on language-based tasks like summarization, text generation, classification, and more. Microsoft's investment in OpenAI and the integration of GPT into the Bing search engine highlight the significance of these language models.
The next wave of text-based machine learning models relies on self-supervised learning and aims at making accurate predictions.
Scientific, Healthcare and Medical Contributions
Generative AI is a game-changer in drug discovery and healthcare. It helps create new proteins for antibodies, vaccines, and gene therapy, and automates tasks like medical coding and imaging. In life sciences, it speeds up research by designing synthetic genes for various applications, like creating biological pathways and optimizing gene expression. This AI also generates synthetic patient data for training AI models and simulating clinical trials.
Generative AI is making waves in the pharmaceutical industry, particularly in drug compound discovery, generating candidate molecules and accelerating the development of new drugs. The potential impact on the pharmaceutical and medical-product industries is significant, with estimated annual revenues ranging from $60 billion to $110 billion (Source: McKinsey). This reflects the resource-intensive nature of drug discovery, where improving the speed and quality of research can generate substantial value, especially when tasks can be completed in weeks, compared to several months using traditional techniques.
Implementing generative AI could increase sales productivity by approximately 3 to 5 percent of current global sales expenditures. *
* McKinsey
Navigating Challenges and Ethical Considerations
Despite their potential, these generative AI tools pose issues like accuracy, trustworthiness, bias, hallucination, and plagiarism.
The convincing realism of generative AI content brings new risks, making it challenging to detect AI-generated results and identify errors. This poses significant problems, especially in:
- the creation and spreading of deepfakes, leading to misinformation
- assessing issues like copyright infringement or the reliability of sources.
The ethical use of this technology is under scrutiny, with worries about data leakage and privacy breaches. Ethical implications also extend to generative AI art, where the reimagining of artists' works raises questions about originality and credit.
Moreover, the environmental impact of generative AI is a growing concern due to the extensive processing power required for training models, consuming substantial energy.
Generative AI's misuse is recognized globally, prompting discussions at the United Nations Security Council about both its positive and harmful impacts.
Source: Techopedia
The Future of Generative AI
The trajectory of generative AI has been characterized by scaling up models for better results, with the size correlating with improved performance. Contrary to this trend, smaller models focused on specific domains have proven to outperform larger, general-purpose models in certain contexts.
Looking ahead, generative AI is prepared to reshape many industries with varying impacts. Content creation jobs will transform into content editing roles, requiring different skill sets. The workforce's interaction with applications will become more conversational and proactive, altering the traditional user experience. Evolution in translation, drug discovery, anomaly detection, and content generation are expected.
While predicting the exact impact is challenging, the ongoing integration of generative AI into various tasks necessitates a reevaluation of human expertise in an automated and augmented landscape.
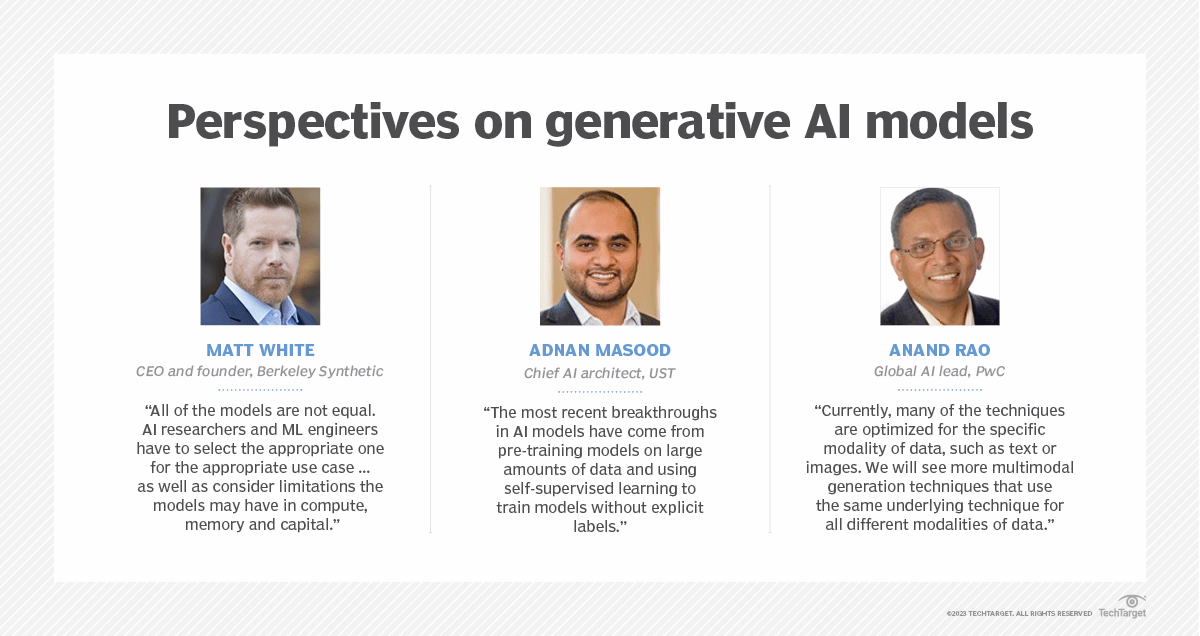
Source: TechTarget
Conclusion: Embracing Opportunities and Managing Risks
The journey through Generative AI extends beyond text, video, and images, finding applications in music, game development, and healthcare. It's an exciting, evolving field, offering opportunities for those who delve into its early stages. The future promises further integration into our daily lives and industries.
While generative AI offers vast potential, the evolving landscape requires staying informed about regulatory changes.
The allure of innovation is captivating, but the path forward is not without challenges or risks!